If LLMs are "human wisdom", then AI Agent is "using fire and flint" to lead human civilization into the next era.
In 2024, AI Agent is the hottest concept. On the one hand, the big model track has cooled down and presented a winner-takes-all situation; on the other hand, AI Agent is the best form of big model application, which can solve the limitations of LLMs in specific application scenarios.
So, what is the current status of AI Agent adoption? Which fields will emerge first?
We combined two important reports, the State of AI Agents released by LangChain and the 2024 State of AI Agents released by Langbase, to try to find the key issues in the development and adoption of AI Agents.
LangChain surveyed more than 1,300 professionals, including engineers, product managers, business leaders and executives; industry distribution: technology (60%), financial services (11%), healthcare (6%), education (5%), consumer goods (4%). Report link https://www.langchain.com/stateofaiagents
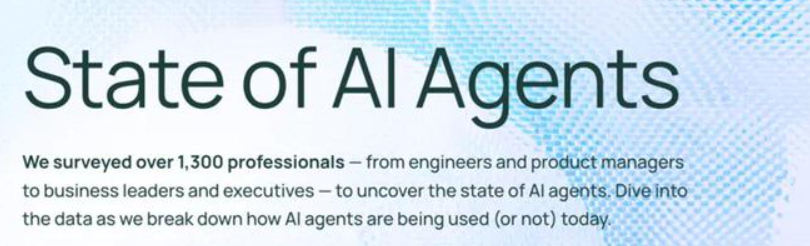
Langbase surveyed more than 3,400 professionals (over 100 countries), including C-level executives (46%), engineers (26%), customer support (17%), and MKT (8%). Report link: https://langbase.com/state-of-ai-agents
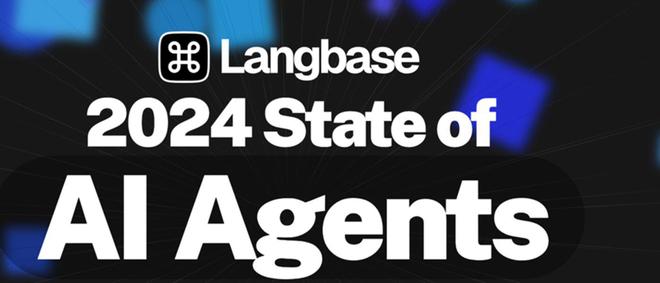
The specific contents are as follows:
1. Who is using AI Agents?
The LangChain survey shows that about 51% of respondents have already adopted AI Agents in production environments; and 78% of respondents plan to introduce AI Agents into production applications in the near future.
In terms of size, medium-sized enterprises with 100-2000 employees are the most active, with an adoption rate of 63%; in terms of industry, 90% of non-tech companies have deployed or plan to deploy AI Agents, which is comparable to technology companies (89%).
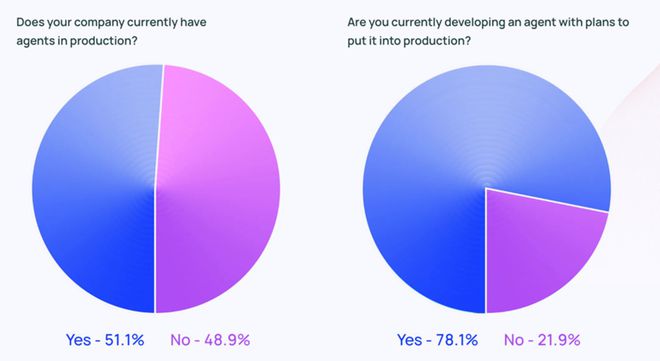
Langbase survey shows that currently, the proportion of experimentation uses of AI (non-AI Agent) is far greater than that of production uses, although the latter is steadily increasing.
.jpg)
2. Which AI Agent base model is the best?
Langbase survey shows that OpenAI (76%) is dominant, Google (59%) is rapidly rising and becoming its strong competitor, followed by Anthropic (47%). Meta's Llama, Mistral and Cohere are not very influential, but their growth momentum cannot be ignored.
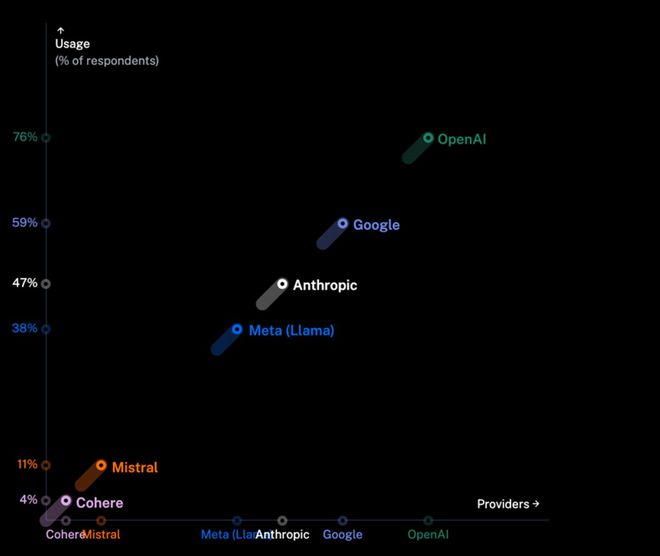
The adoption of each major model is as follows:
- OpenAI is a leader in technology and marketing applications, and is also an expert in translation;
- Google performed quite well in the fields of health and translation, demonstrating its strong capabilities in language and medicine;
- Anthropic is a master at handling technical tasks, but is less used in marketing and translation;
- Mistral performs well in technology and science, but is a partial student;
- Meta is widely used in technology and science;
- Cohere is making progress in multiple areas.
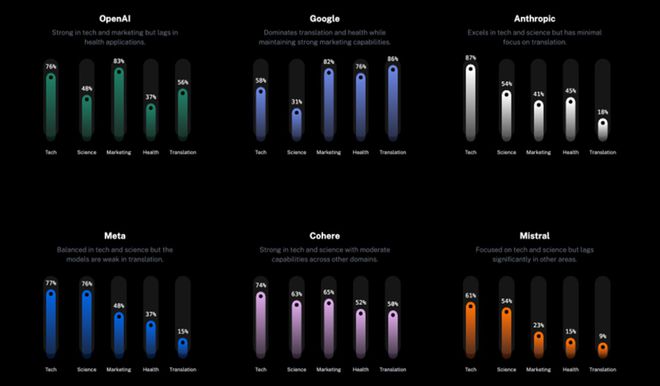
3. What factors influence the selection of AI Agent base model?
Langbase survey shows that performance(45%) is the most important factor , followed by security (24%) and customizability (21%), and cost (10%) has a relatively small impact. (Note: This is basically the same as "What are the concerns of enterprises in adopting AI Agents")
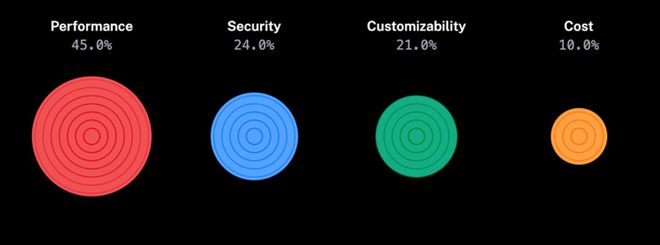
4. In what scenarios do enterprises use AI Agents?
LangChain survey shows: research and summarization (58%), personal assistance / productivity tasks (53.5%), customer service (45.8%).
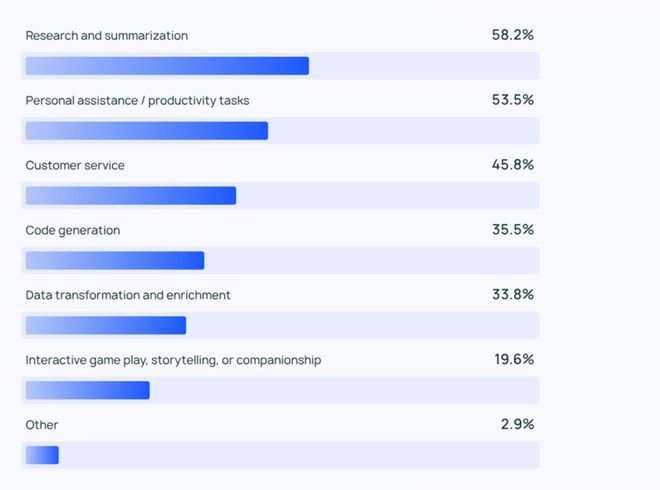
The results show that people want to delegate time-consuming tasks to AI Agents.
- Knowledge filter : AI Agent can quickly extract key information. In literature review or research analysis, people do not need to manually filter massive amounts of data;
- Productivity accelerator : AI Agent can help arrange schedules, manage tasks, improve personal efficiency, and allow people to focus on more important work;
- Customer service assists : AI Agent helps companies handle customer inquiries and solve problems more quickly, greatly improving the team's response speed.
The Langbase survey shows that automation and simplification are the primary goals of enterprises in adopting AI , and they benefit in terms of efficiency and process simplification; customized solutions and enhanced collaboration capabilities reflect the growing flexibility of large models and consumers' interest in shared access to systems.
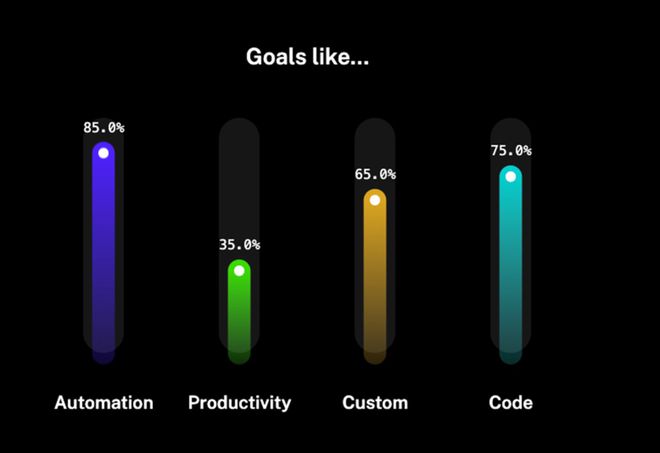
In specific scenarios, the Langbase survey shows :
- AI big models are most widely used in software development (87%) ;
- Next are text summarization (59%), marketing (50%), IT operations (48%), and customer service (43%);
- Finally, there are areas such as human resources (26%) and legal compliance (15%) .
It is worth noting that this result is somewhat different from the above-mentioned LangChain survey. The main reason is that Langbase’s questioning method is an AI big model, not an AI agent.
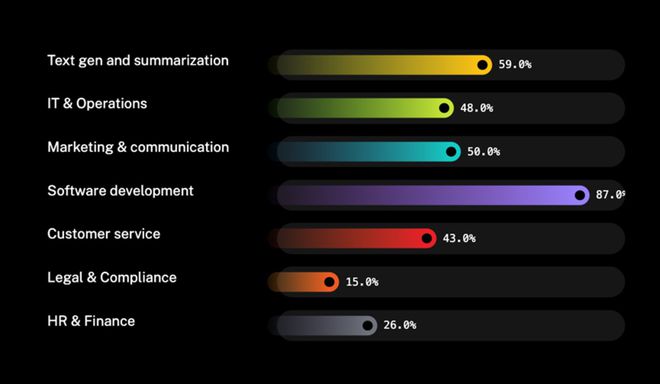
5. What are the concerns about using AI Agent in production?
The LangChain survey shows that performance quality (41%) is the primary concern, far outweighing factors such as cost (18.4%) and security (18.4%).
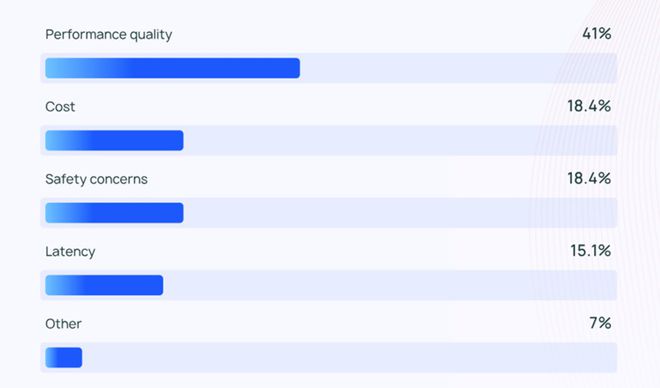
AI agents rely on LLM “black box” control workflows, which introduces unpredictability and increases the risk of error. As a result, it is difficult for teams to ensure that their agents can always provide accurate and contextual responses.
Performance quality is particularly important for small businesses - 45.8% of small businesses list performance quality as a major concern, while cost factors are only 22.4%.
For medium and large enterprises that must comply with regulations and handle client data sensitively, security concerns are also prevalent and outweigh cost factors.
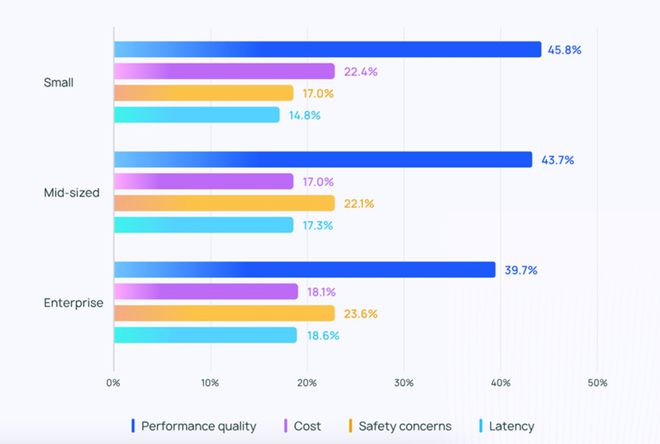
In addition to the above factors, LangChain found in its written response that the team also faced knowledge and time difficulties.
- Insufficient knowledge : Many teams lack the professional skills to build and deploy AI agents, especially in specific application scenarios. Employees also need to work hard to hone their professional skills to use AI agents efficiently.
- Limited time : Building a reliable AI agent requires a lot of time, including debugging, evaluation, and model fine-tuning.
The Langbase survey shows that complex expansion and deployment processes are the primary issues hindering adoption; followed by data privacy and security compliance; lack of monitoring tools and high infrastructure costs also hinder the implementation of technology.
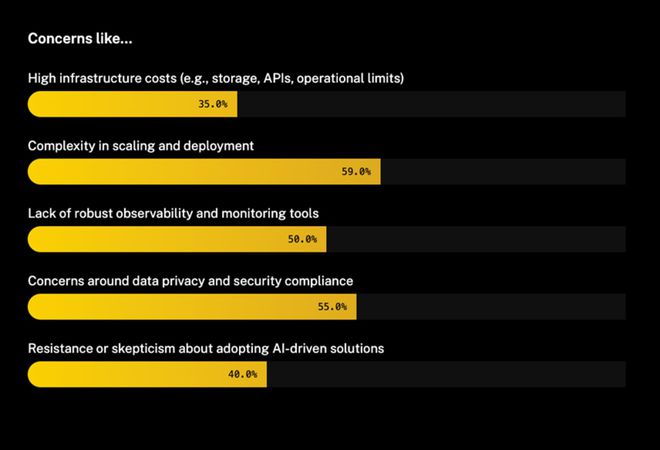
6. What are the issues related to AI Agent development?
Langbase survey shows that deploying LLM and AI Agents in production environments faces key challenges, including high customization difficulty, lack of evaluation methods for quality assurance, and insufficient reusable infrastructure. Fragmented tools, integration issues, and scalability limitations further exacerbate the difficulties, highlighting the need to build simplified processes and powerful supporting tools.
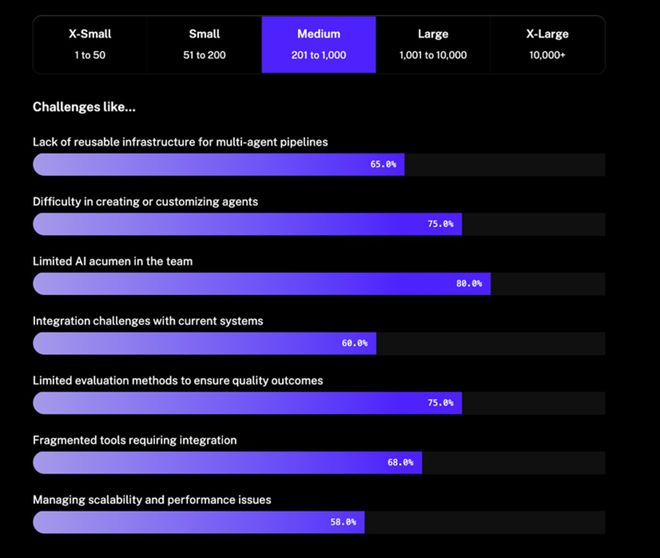
Developers consider AI Agent version control to be the most important feature of the development platform. Powerful SDK, library ecosystem, and local development environment are also of interest.
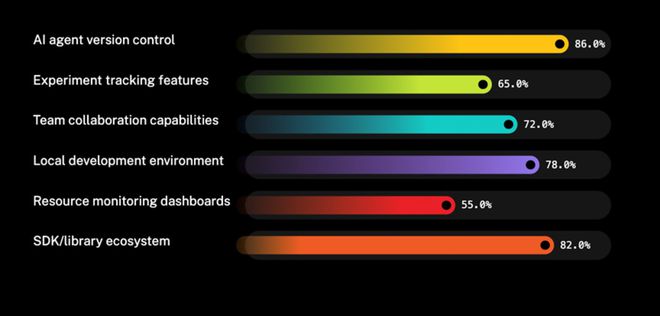
Most respondents require multi-agent RAG capabilities to improve contextual information processing; evaluation tools are also important to ensure that AI systems work as expected; multi-agent pipelines are also a key technology to implement complex tasks in production.
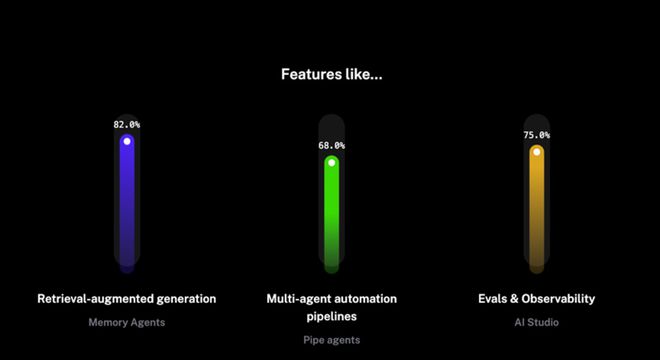
7. What are your prospects for AI Agent?
LangChain survey shows that when enterprises adopt AI Agents, they have new expectations but also face continuous challenges.
New expectations:
- Managing multistep tasks : AI agents have deeper reasoning and context understanding capabilities and can handle complex tasks.
- Automating repetitive tasks : AI agents are seen as a key tool for automating routine tasks, freeing up human resources for more creative work.
- Task routing and collaboration : Optimize task allocation to ensure that the right agent handles a specific problem at the right time, especially in multi-agent systems.
- Human-like reasoning : Unlike LLMs, AI Agents can trace back and optimize their decisions and adjust strategies based on new information, similar to the human thinking process.
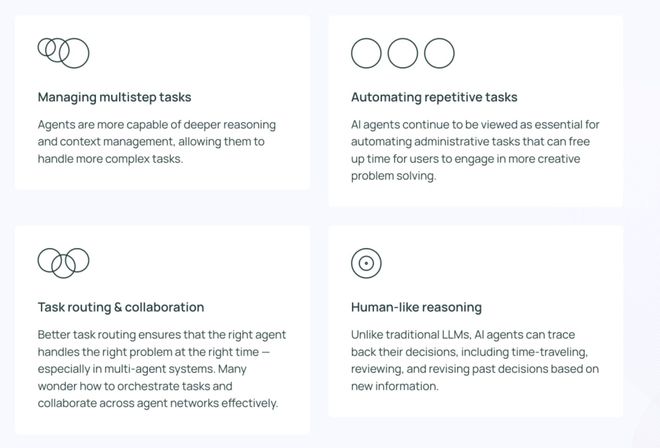
Main challenges:
- Black box mechanism of the agent : Engineers found it difficult to explain the functions and behaviors of the AI agent to their team and stakeholders. Although the visualization step helped to understand what was happening, the internal mechanism of the LLM was still a black box, which made it more difficult to explain.
Focus:
- Excitement for open source AI agents : Many people have shown great interest in open source AI agents, believing that collective intelligence can accelerate innovation.
- Anticipation for more powerful models : We expect more advanced AI agents, driven by more powerful models, to handle complex tasks with higher efficiency and autonomy.
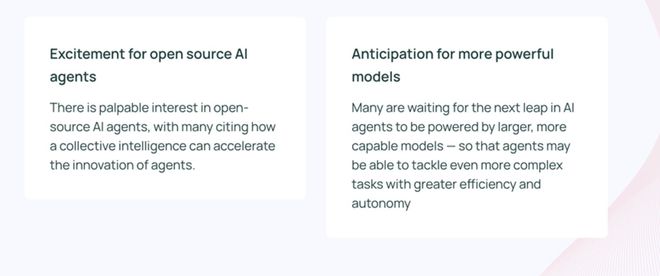
Conclusion
Judging from the willingness of enterprises to adopt, 2025 may become the first year of the explosion of AI Agent.
From the perspective of explosive growth areas, software development, customer service, marketing and other fields will be the first to produce seed players. Currently known ones include the programming artifact Cursor, the "ancestor" of AI Coding Replit, and so on.
It is worth noting that both reports reveal the biggest difficulty in the current implementation of AI Agents - accuracy , and even the cost factor ranks second or third.
One way is to wait for the emergence of the "supreme" model, but this depends on the capabilities of the giants. To some extent, the emergence of AI Agent itself is to solve the limitations of LLM in specific application scenarios . Therefore, developers need to work as hard as possible on other technology stacks such as memory, planning, and tool use.
If this cannot be achieved quickly, we can also change our thinking: an AI agent that is commercially successful in the short term is not necessarily a product that appears to be the most "agent-like"; rather, it is a product that can balance performance, reliability, and user trust.
In other words, if full autonomy is not possible, developers need to consider how to integrate human employees from the beginning to ensure absolute accuracy.
As Andrew Ng said, the adjective "Agentic" can help us better understand the nature of this type of intelligent body than the noun "Agent". Just like the L1-L4 of self-driving cars, the evolution of Agent is also a process. Perhaps, a definite trend is to have AI Copilot first, and then AI Agent. However, the word Copilot may become less noticeable and replaced by "a small amount of Agentic capabilities".